The Power of Contrasts in Science Education

Contrasts of any sort structure attention. Bring a handful of similar — but different — cases together and we look at things differently. Seeing the cases together provides two primary benefits.
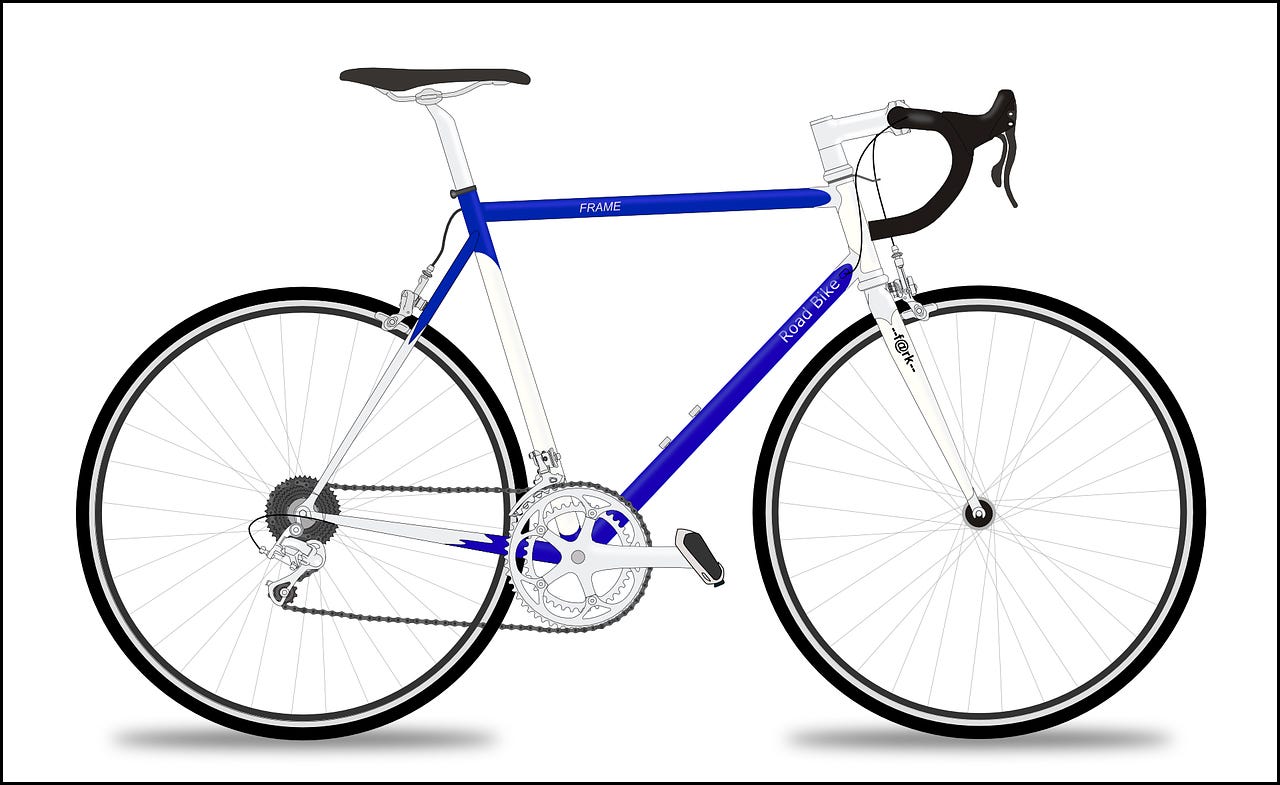
First, students can see details of the cases that they would have missed otherwise. See a photo of a bicycle and it just looks like a bicycle: there’s the main frame, two wheels, the gear mechanism, and the handlebars. But see two bicycles side-by-side and the differences become more pronounced. Those aren’t just any wheels: those are big wheels. They have a thick tread. The gear mechanism is simpler. Certain details of the bicycles have become more pronounced.
The second benefit of contrasting cases comes from seeing structure in the variation. Bicycles can come in a wide variety of shapes and sizes. Appropriately chosen cases can illustrate relationships between, say, the ratio of the size of the gears and the mechanical advantage of the bicycle. Beyond merely noticing important details, research suggests that contrasting cases can prepare students to learn about deeper reasons that explain that variation.

Science classes are full of opportunities to use contrasting cases. Teach anatomy? Contrasting cases of bones provided a great way to notice important details. Teach geology? Contrasting cases can be a great way of introducing students to variation in rock types. But the value of contrasts goes beyond teaching science content.
Contrasts are great for framing productive arguments.
Science is fundamentally about explaining. It’s about arguing with evidence, constructing possible explanations, and proposing ways of evaluating these explanations. If you’re interested in teaching students to think like scientists — if you’re interested in teaching them what science is and not just feeding them scientific content — contrasts offer tremendous value in the science lab and in the science classroom.
Consider the traditional science lab. I took college-level chemistry three times. On lab days, we would walk in, get in our groups, and get the experimental procedure. It was a recipe for how to perform the experiment. Go to the side table, measure out 5 grams of sodium chloride. Then measure out 12 ounces of hydrogen dioxide. The best part was holding the cool glass beakers. The experiment usually demonstrated something about the ideas we were learning in class, although even at the time I would be hard-pressed to say what.
Did I learn much about how science worked? No. Did the experience help me learn the content I was learning in lecture? Research tells us probably not. This kind of approach to science labs — the recipe approach — doesn’t involve meaningful contrasts and doesn’t support productive arguments. You either got the expected answer or you didn’t. If you didn’t, you probably screwed up somewhere. Your conclusion is a list of all of the ways you may have screwed up. Lab over. If you got the expected answer, then good for you — no further discussion necessary.
Traditional science labs offer only a superficial mimicry of science. What they introduce students to is the front-stage of science: the completed, conceptualized experiment that, when performed correctly, demonstrates some important principle. They give students little to no practice in scientific decision-making. If argument is central to science, as some have suggested, these recipes provide few opportunities for argument. If model-based reasoning is central to science, as others have suggested, these recipes provide few opportunities for model building. They just don’t create environments for productive science talk.
But contrasts can.
Consider the following three situations:
1. A student determines whether data is consistent with a given hypothesis
2. A student evaluates two contrasting explanations for a phenomenon
3. A student evaluates two conflicting data sources purporting to represent a phenomenon.
Situation one involves no contrasts. There is some room for argument here — how close is close enough to say that the evidence is consistent with the hypothesis? And there is some room for teaching important practices in science: most data needs to be statistically analyzed to learn much. But simply determining whether data is consistent with a hypothesis leaves little opportunity for rich scientific thinking.
But consider the story of the discovery of Neptune. By the 1830s, astronomers realized there was a problem with Uranus. Predictions of the planet’s orbit (based on Newtonian physics) did not match up with observations of where the planet actually seemed to be. Saturn and Jupiter didn’t have that problem, but Uranus did.
Determining that these observations were inconsistent with Newtonian physics was the easy part (at least relatively easy — it required a fair number of painstaking calculations without modern technology). The interesting question is: what to do about it? Throw out Newtonian physics? No, at least not entirely. But perhaps Newton’s description wasn’t entirely accurate. Was a previously unidentified planet disturbing Uranus’s orbit? Possible, but astronomers had been using powerful telescopes for 200 years — how could they have missed it? Measurement error? Also possible, but, the measurements for Saturn and Jupiter didn’t have the same problem.
Now we are in the world of contrasting explanations. Of course, the correct explanation turned out to be (b): a previously unidentified planet, later named Neptune, could account for Uranus’s orbital mismatches. But determining that required thinking about possible explanations and picking the best one. Which explanations are the most plausible, and why? What kind of evidence would distinguish one explanation over another? What’s feasible to do?
The explanations for the mismatch in Uranus’s orbit were quite different from one another. Explanations that are very similar — yet still distinct — offer a more direct comparison to contrasting cases. Take the single-celled organism known as Euglena. Euglena respond to light. They have photo-receptors that recognize light (call them eyes) and Euglena tend to move towards light. Engineers and educators have built several systems for students to interact with Euglena in real-time. These tools can be used in various ways.
Here’s a question without any contrast: “Is the Euglena behavior you witness consistent with the hypothesis that they have an eye?” The evidence that you see can be:
1. Consistent with the model
2. Inconsistent with the model
Figuring out whether the evidence is consistent with this hypothesis is pretty straightforward. Do Euglena respond to light or color in some way? If so, seems like they have an eye. If not, then probably not.
Here is a question with a contrast: “Which model of Euglena is more consistent with the behavior you witness: a one-eye model or a two-eye model?” Now the evidence can be:
1. Consistent with both models
2. Inconsistent with both models
3. Consistent with the one-eye model, but inconsistent with the two-eye model
4. Consistent with the two-eye model, but inconsistent with the one-eye model
Both models predict that the Euglena will respond to light, but each model predicts a slightly different “how”. Teasing out the kinds of experiments or observations that would let us distinguish one model from the other is quintessential scientific practice. It has the added benefit that to do so requires thinking deeply about content knowledge — how unicellular organisms move.
But it’s hard to think through the implications of each model. For many students, just thinking about it isn’t enough. The teacher must adopt the role of a discussion facilitator, rather than simply be a judge of correctness. Well-designed technology can also make the implications of each model more apparent. Students, for example, might be able to watch simulations of each model, which can lead to insight about the relationship of each model to the organisms’ predicted behavior.

Contrasting models, however, are not the only productive contrasts. Scientists often draw on multiple data sources when making observations and exploring possible explanations. When these data sources conflict with each other they have to determine which is a better representation of what’s actually going on.
Volunteers in the citizen science project Eterna were faced with just such contrasts. In the simulation, RNA molecules seemed to be fold in one way, but when the same designs were tested in an actual lab, something quite different seemed to be happening. Which to believe? And why?
Resolving these questions requires investigating (and arguing about) the processes that generate the data. The instinct, at least for most volunteers and developers, was to believe actual lab results over results coming from a simulation. After all, the whole point of the project was to improve upon existing models of RNA folding through open lab experiments. But the lab results had to measure molecular structure indirectly. There was no camera to take a snapshot of the molecule all at once. And the indirect measures introduced bias that has to be accounted for. Sometimes, the simulation was right.
The contrast became a conversation: how is the lab currently measuring the structure of the molecule? What does the simulation do to simulate molecular structure? When should we believe one over the other?
These kinds of conversations occurred over and over again as volunteers confronted new contrasts. Why do algorithmic designs fail in different ways than human designs? To answer that, we have to know something about how the algorithms work and something about how humans go about molecular design. Why do these two lab measurements seem to conflict? To answer that, we have to know something about how each lab measurement is produced. Contrasts keep students on the hook. Asking them if they got the expected answer does not.
Contrasts: they’re not just for learning bird species and bicycle types. Use them to structure productive science talk. Use them to engage students in scientific practice.
Member discussion